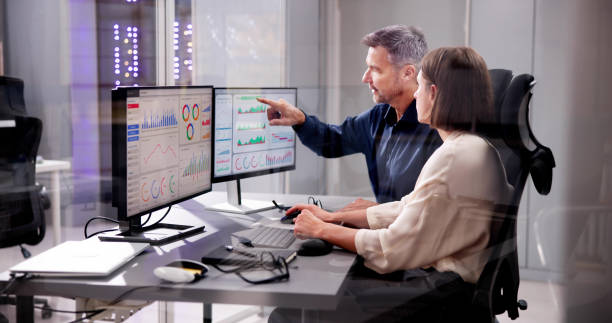
- January 29 2025
- SFI Solution Team
In the age of digital transformation, organizations are progressively depending on data-driven decision-making. Predictive analytics, an essential aspect of advanced data science, is transforming the management of integration workflows within businesses. By utilizing historical data, machine learning algorithms, and statistical models, predictive analytics improves efficiency, minimizes errors, and simplifies intricate integration processes. This article examines the impact of predictive analytics on integration workflows and emphasizes the necessity for businesses to embrace this technology to achieve sustainable success.
Understanding Predictive Analytics in Integration Workflows
Predictive analytics uses historical and real-time data to forecast outcomes and optimize processes. In integration workflows, this translates to the proactive identification of bottlenecks, improved data mapping, and automated decision-making. By integrating predictive analytics into workflow automation tools, businesses can achieve real-time monitoring and intelligent process optimization.
Key Benefits of Predictive Analytics in Integration Workflows
-
Enhanced Efficiency Predictive analytics identifies workflow inefficiencies by analyzing patterns and trends. By predicting potential failures and optimizing processes, organizations can reduce downtime and increase operational efficiency.
-
Proactive Error Detection and Resolution Traditional integration workflows often face data inconsistencies, missing fields, and transformation errors. Predictive analytics flags anomalies before they disrupt operations, enabling teams to take preventive actions.
-
Data-Driven Decision-Making Businesses can leverage predictive insights to optimize data integration strategies. By understanding data trends, organizations can make informed decisions on system updates, API integrations, and third-party tool adoption.
-
Cost Reduction Predictive models help in reducing redundant processes, optimizing resource allocation, and minimizing manual intervention. This leads to significant cost savings in IT operations and integration management.
-
Scalability and Future-Readiness As organizations scale, integration workflows become more complex. Predictive analytics ensures seamless expansion by forecasting integration challenges and automating scalability solutions.
Use Cases of Predictive Analytics in Integration Workflows
1. Intelligent API Management
Predictive analytics optimizes API performance by analyzing historical request-response times and predicting potential failures. Organizations can preemptively scale resources and prevent downtime.
2. Optimized Data Transformation
Data transformation processes involve mapping, cleaning, and validating data. Predictive models suggest optimal transformation rules, reducing manual effort and enhancing data quality.
3. Automated Workflow Orchestration
Predictive analytics enables dynamic workflow automation by anticipating process dependencies and optimizing task execution. This ensures seamless system integrations across multiple platforms.
4. Fraud Detection and Security Enhancement
Predictive algorithms detect irregular patterns in data transfers, preventing security breaches and fraudulent activities within integration workflows.
5. Predictive Maintenance in IT Infrastructure
IT teams can use predictive analytics to anticipate server failures, network downtimes, and integration issues, ensuring uninterrupted business operations.
Implementing Predictive Analytics in Integration Workflows
Step 1: Data Collection and Preparation
Gather structured and unstructured data from various integration points. Ensure data quality by eliminating duplicates and inconsistencies.
Step 2: Model Selection and Training
Choose suitable machine learning models based on business needs. Train models using historical data to identify trends and predict workflow inefficiencies.
Step 3: Integration with Workflow Automation Tools
Embed predictive analytics within workflow automation platforms, such as ETL (Extract, Transform, Load) tools, RPA (Robotic Process Automation), and API management solutions.
Step 4: Real-Time Monitoring and Adjustments
Continuously monitor predictive insights and refine models to enhance accuracy. Implement feedback loops for ongoing process optimization.
Step 5: Scalability and Continuous Improvement
As business requirements evolve, update predictive models to adapt to new data patterns and integration complexities.
Challenges and Considerations
Despite its advantages, implementing predictive analytics in integration workflows presents challenges, including:
-
Data Privacy and Compliance : Ensuring data security and regulatory compliance (e.g., GDPR, CCPA) is crucial.
-
Model Accuracy and Bias : Predictive models must be regularly updated to minimize bias and inaccuracies.
-
Technical Complexity: Organizations must invest in skilled professionals and infrastructure for successful implementation.
Conclusion
Predictive analytics is revolutionizing integration workflows by facilitating proactive decision-making, minimizing errors, and enhancing efficiency. Organizations that adopt predictive analytics can attain smooth integrations, improved scalability, and more economical operations. As companies persist in their innovation efforts, the incorporation of predictive analytics into workflows will serve as a pivotal factor, providing a competitive advantage in the rapidly changing digital environment.
Are you ready to optimize your integration workflows with predictive analytics? Start your journey today and stay ahead of the competition!
Previous Post